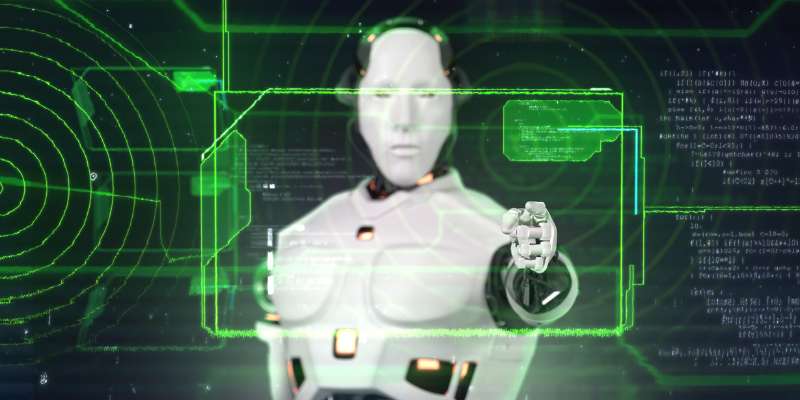
Understanding AI Algorithms Behind Robo-Advisors
Understanding AI Algorithms Behind Robo-Advisors
Introduction
The world of investing has undergone a seismic shift, thanks to AI-powered investment platforms. These platforms, often referred to as robo-advisors, have democratized access to professional-grade financial advice, making investing easier and more efficient. But what powers these robo-advisors? It’s the sophisticated AI algorithms working behind the scenes to optimize portfolios and provide personalized financial guidance. This article will delve into the fascinating world of AI in robo-advisors, breaking down the technology and its implications for investors worldwide.
Table of Contents
What Are Robo-Advisors?
Robo-advisors are digital platforms that provide automated, algorithm-driven financial planning services. They analyze user inputs such as income, financial goals, and risk tolerance to recommend investment strategies. Unlike traditional financial advisors, these platforms are cost-effective and accessible 24/7, making them ideal for modern investors.
Key features of robo-advisors include:
- Automated portfolio management
- Low fees compared to human advisors
- Goal-based financial planning
- Continuous monitoring and rebalancing of portfolios
The Role of AI in Robo-Advisors
AI is the backbone of robo-advisors, enabling them to process massive datasets and make precise decisions. By leveraging technologies like AI sentiment analysis for investments, these platforms can assess market trends and predict asset performance. AI’s role goes beyond data crunching; it ensures that each user receives tailored advice, making financial planning more efficient and effective.
Capabilities powered by AI include:
- Predictive analytics for market forecasting
- Behavioral pattern recognition to understand investor preferences
- Natural language processing (NLP) for user-friendly interactions
Types of AI Algorithms Used in Robo-Advisors
- Machine Learning Algorithms: Machine learning enables robo-advisors to improve their decision-making over time. These algorithms analyze historical market data to identify patterns and predict future trends.
- Natural Language Processing (NLP): NLP powers chat interfaces and makes financial advice accessible to non-experts. It allows users to communicate their goals and preferences in plain language.
- Reinforcement Learning: This type of AI adapts to changing market conditions by continuously optimizing investment strategies based on feedback loops.
- Predictive Analytics Algorithms: These algorithms forecast asset performance and market trends, ensuring that portfolios align with future opportunities.
How Robo-Advisors Optimize Portfolios
At the heart of robo-advisors is portfolio optimization, a process driven by AI algorithms. These platforms integrate Modern Portfolio Theory (MPT) with AI to create diversified, low-risk portfolios.
Steps in portfolio optimization:
- Risk Assessment: AI analyzes user data to determine risk tolerance.
- Asset Allocation: Diversification strategies are implemented based on risk profiles.
- Rebalancing: Portfolios are automatically adjusted to maintain optimal performance.
Personalization in Robo-Advisors
One of the standout features of robo-advisors is their ability to personalize investment strategies. By analyzing demographic, financial, and behavioral data, AI tailor’s recommendations to meet individual goals.
Benefits of AI-driven personalization:
- Customized asset allocations
- Dynamic goal adjustments based on life changes
- Enhanced user engagement and satisfaction
Benefits of AI Algorithms in Robo-Advisors
The adoption of AI in robo-advisors has revolutionized investing. Here’s why:
- Accessibility: Platforms like AI democratizing investment advice have brought financial planning to the masses.
- Cost Efficiency: Lower fees compared to traditional advisors.
- Precision: Data-driven insights reduce the risk of human error.
- Scalability: AI can handle a large volume of users without compromising quality.
Challenges and Limitations of AI in Robo-Advisors
Despite their advantages, robo-advisors face several challenges:
- Transparency Issues: Many users struggle to understand the algorithms’ decision-making processes. Greater transparency is needed to build trust.
- Data Privacy and Security: Robo-advisors collect sensitive financial and personal data, raising concerns about breaches.
- Ethical Considerations: Biases in AI models can lead to inequitable investment strategies.
- Market Volatility: During periods of high market volatility, the performance of robo-advisors can be inconsistent.
Real-World Case Studies of Successful Robo-Advisors
- Wealthfront: Wealthfront uses AI to automate tax-loss harvesting, optimizing portfolios for tax efficiency. Its AI-powered planning tools have set a high standard in the industry.
- Betterment: Betterment excels in goal-based investing, leveraging AI to provide personalized financial plans. It continuously monitors portfolios to align with user objectives.
Lessons from these platforms highlight the importance of AI in enhancing user experiences and outcomes.
The Future of AI in Robo-Advisors
The future of robo-advisors is bright, with advancements promising even more robust capabilities. Here’s what to expect:
- Enhanced Predictive Capabilities: Improved AI in stock market predictions will lead to more accurate forecasts.
- Blockchain Integration: For secure and transparent transactions.
- Global Adoption: AI-driven platforms will expand access to underbanked regions, promoting financial inclusion.
Conclusion
AI has transformed robo-advisors from a niche service to a mainstream financial tool. With advancements like AI democratizing investment advice, these platforms are set to redefine investing for generations to come. Understanding the algorithms behind them empowers investors to make informed decisions and embrace the future of financial planning. The journey has just begun, and I’m thrilled to see where this technology will take us next.