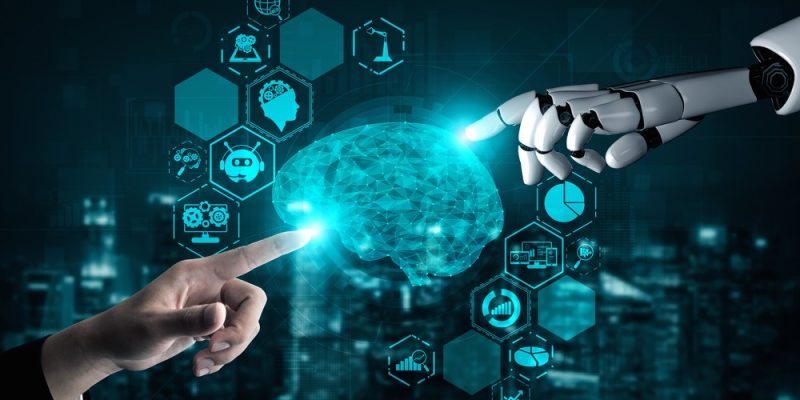
Can AI and Machine Learning Simulate the Human Brain?
Artificial Intelligence (AI) and Machine Learning (ML) have been making significant strides in technological advancements in recent years. The field has come a long way since Alan Turing introduced AI and Deep Blue defeated Garry Kasparov in a chess match. However, the question remains: can we create machines that can replicate the workings of the human brain and human intelligence?
AIHBrain Model
Simulating the Human Brain The AIHBrain model is a promising development that can help us understand how the human brain works. The model comprises six key components: problem formalization, critic component, historical databases, planning component, parallel execution component, and scheduling component. The deep cognitive neural network (DCNN) is the underlying technology that enables the AIHBrain model to simulate human brain function. While we are still far from achieving general AI, we are getting one step closer to creating a model that can accurately simulate the human brain.
What is AI?
For those new to the field, AI refers to the simulation of human intelligence by intelligent machines, often in the form of computer systems. ML is an essential component of AI that enables computers to learn and make predictions without human intervention.
Simulating the Human Brain
So how close are we to simulating the workings of the human brain with AI technology? The answer is that we have made significant progress. Scientists at universities in the US and abroad have developed neuromorphic computing models that mimic the brain’s structure and functions. These breakthroughs have been made possible, in part, by the development of brain-computer interface technology.
Implications for Artificial Intelligence
The ability to simulate the human brain with AI technology has far-reaching implications. For instance, the technology can help us develop intelligent machines that can understand natural language, recognize images, and make decisions autonomously. It can also help us create more efficient and effective robots that can learn and adapt to new situations.
Read Also: AI in Home Robots: How is it Used?
AIHBrain: Revolutionizing Artificial Intelligence with Brain-Inspired Machine Learning
In recent years, machine learning has seen tremendous growth, and its applications have been observed in various fields. However, current machine learning models are limited by their ability to process and interpret data accurately. The development of AIHBrain – a novel, brain-inspired machine learning framework – is set to revolutionize the field of artificial intelligence.
What is AIHBrain?
AIHBrain is a machine learning model that mimics the way neuronal cells work in the human brain. By simulating human brain intelligence, AIHBrain has the potential to transform the way deep learning models are developed and artificial intelligence is trained. With this novel approach, machines can analyze objects and ideas and apply reasoning just like humans.
Overcoming Current Challenges
One of the significant challenges of current machine learning models is their limited ability to learn and interpret data accurately. While some models produce inconsistent results, others are challenging to interpret due to their one-dimensional programming. By imitating the inner workings of the human mind, AIHBrain can overcome these limitations.
Applying Human-like Intelligence
The AiHBrain model applies three basic layers: data input, processing, and data output. The data input layer receives data from all sources and channels. The data processing layer then applies several human-like intelligent approaches to select or create the most appropriate model for the analysis. The technology takes into account any existing knowledge-based systems and historical data like humans would. It may also adapt existing algorithms to suit the new task. Finally, the data output layer showcases the findings produced during the previous stage.
A Toolbox of Machine Learning Models
AIHBrain has access to a data archive, pre-existing knowledge, and a range of machine learning models to choose from. It also has the capability to select the most suitable tool for a given problem. This skill is comparable to a person using human intelligence to select the right tool from a toolbox.
Future Applications of AIHBrain
The innovation and application of AIHBrain are already becoming evident in products like self-driving cars. However, the technology’s future development options include autonomous weapons and other types of intelligent machines.
AIHBrain Fundamental Architecture: A Detailed Look at Its Infrastructure
As the field of artificial intelligence continues to advance, so do the models that power it. One such model is the AIHBrain, which boasts a more intricate architecture than traditional models. In this article, we will explore the various components that make up the AIHBrain’s infrastructure and how they work together to solve previously unknown problems.
Problem Formalization Component: Putting Data into Context
At the heart of the AIHBrain’s data input stage is the problem formalization component. This component is critical in that it puts mixed data from different sources into context. The additional real-world data from the system’s meta-world container helps to add more meaning to the input data. Think of the meta-world container as the model’s history component, providing context to the input data.
Lastly, the input data is combined with a task objective. Together, these three components hold all the necessary information for a complete analysis. If any of them are missing or incomplete, the output may be compromised.
Critic Component: Qualifying and Generating Requirements
Another crucial component of the AIHBrain’s infrastructure is the critic component. It consists of two parts: the data enhancer and the requirement generator.
The data enhancer adds previously existing information to complement the new input. It also applies qualifications and puts constraints on the new data to ensure its accuracy and relevance. This ensures that the data is properly qualified before it is used to make decisions.
The second part of the critic component is the generator of requirements. This component generates the necessary requirements that the intermediate data output needs to meet. These requirements ensure that the data output is relevant and accurate, making it useful for making informed decisions.
The Orchestrator Component: Understanding AiHBrain’s Model Framework
If you’re looking for a cutting-edge AI model framework, the AiHBrain should be on your radar. This framework is composed of four parts, namely the model selector, problem qualifier, planner, and parallel executor, which work together to enable supervised and unsupervised learning, search algorithms deployment, reinforcement learning, or a combination of these techniques.
Flexibility and Adaptability: AiHBrain’s Standout Features
One of the most notable strengths of the AiHBrain model is its capacity to address multiple issues concurrently, thanks to its human language processing capability. Additionally, it’s highly adaptable and extendable to newly emerging issues, making it a versatile tool for data scientists and researchers.
Fast Convergence: Outpacing Other Frameworks
When it comes to execution time, the AiHBrain model beats other frameworks, thanks to its ability to put machine learning models into context. This speed holds immense potential for future developments, innovations, and applications.
Accuracy: AiHBrain Model’s Precise Outcomes
The AiHBrain model produces more accurate outcomes than other frameworks, as it has the ability to add historical data and world experience to problems. It performs exceptionally well at tasks involving human language and natural language processing, making it ideal for various applications.
Moreover, the framework’s several optimization stages and techniques provide an opportunity to support ensemble learning, making it an even more effective tool for data analysis and machine learning.
Scalability and Availability: The Key to Enhancing AI Frameworks
As artificial intelligence (AI) applications continue to develop, it’s becoming increasingly important to consider scalability and availability when building AI frameworks. With numerous channels already sending data to the framework, the number of channels and amount of data being transmitted is only expected to increase. This is where scalability becomes a critical requirement for any ML framework.
The AiHBrain Model
Subscribers and Publishers To address the need for scalability, the AiHBrain model processes data as a subscriber, while the inputs function as publishers. This approach helps the model manage the increasing amount of data being transmitted to it, without compromising on efficiency.
Empirical Results
Limitations of Current ML Applications Computational cost, high latency, and power consumption are major limitations that hinder the progression of current ML applications, including deep learning algorithms. As the amount of data flowing through these algorithms increases, they require more powerful hardware, which is not a sustainable trajectory. However, by applying human brain intelligence and brain-computer interface technology, we can overcome these limitations.
Deep Cognitive Neural Network (DCNN)
A Revolutionary Model DCNN is a relatively new deep learning model that utilizes characteristics similar to human brain intelligence. With its superior capability for perception, natural language processing, and reasoning, it’s more suitable for neural networks. Moreover, this model can be implemented in an energy-efficient manner, enabling fast decision-making and generalization as part of long-term learning.
DCNN Fast Decision-Making
A Game Changer The DCNN model, when trained using an MNIST dataset, can make decisions 300 times faster than a comparable multi-layer perceptron (MLP) model. This fast decision-making capability is crucial for various AI applications, including autonomous weapons systems.
DCNN Integration With the Reasoning Algorithm
Unleashing the Full Potential When integrated with the reasoning algorithm, the DCNN model shows its true strength. Similar to human brain intelligence, technology is now able to perceive and reason simultaneously. This capacity is critical for innovation and application projects, including autonomous weapons systems. However, the application of brain-based principles reaches much further, with some future development options still unknown.
Framework Based on Neuromorphic Computing Principles
Improved Processing Speed The integration of DCNN with the reasoning algorithm delivers speed when processing high volumes of data, thanks to its framework based on neuromorphic computing principles. This is a significant improvement compared to traditional neural networks.
Read Also:
Conclusion
Scalability and availability are critical requirements for any AI framework. The AiHBrain model uses a subscriber-publisher approach to manage increasing amounts of data without compromising on efficiency. The DCNN model, with its superior capability for perception, natural language processing, and reasoning, can make decisions 300 times faster than comparable MLP models. With its integration with the reasoning algorithm, the DCNN model shows its full potential and ability to perceive and reason simultaneously, unleashing the possibilities for various AI applications.