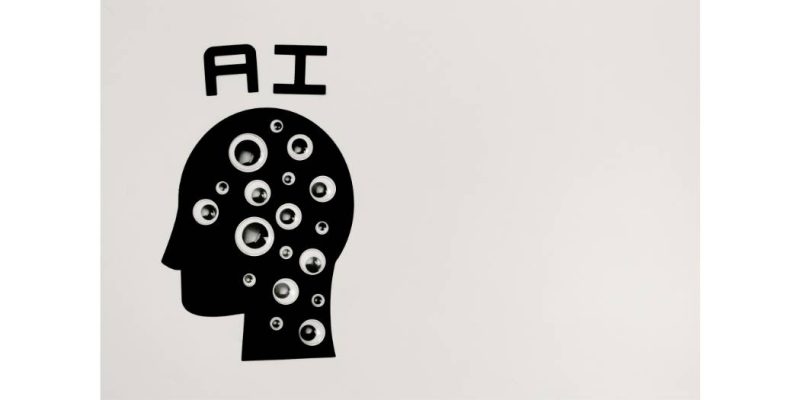
The Ethics of Artificial Intelligence: A Deep Dive
Artificial Intelligence (AI) has become a pivotal part of modern technology, shaping everything from healthcare to entertainment. As AI systems become more advanced and integrated into our daily lives, ethical considerations surrounding their use have become increasingly important.
Understanding these ethical implications is crucial for fostering a responsible and fair AI-driven future. As AI continues to evolve, the ethical challenges it presents become more complex, requiring careful consideration by developers, policymakers, and society at large.
Table of Contents
Understanding AI Ethics
AI ethics primarily revolves around the responsible creation and deployment of AI technologies. It involves ensuring that AI systems operate without causing harm, bias, or unfair advantages. This ethical framework is essential for maintaining public trust and securing the long-term benefits of AI. As AI becomes more autonomous, the responsibility of developers to create systems that align with ethical principles intensifies.
One of the most pressing concerns is the potential for AI to perpetuate or even exacerbate existing biases. Machine learning models, for example, learn from historical data. If this data contains biases, the AI can unintentionally replicate and amplify these biases. This can lead to unfair treatment in areas such as hiring practices, law enforcement, and lending decisions. For instance, if an AI system is trained on data reflecting historical inequalities, such as gender or racial disparities, it may unknowingly propagate these issues, leading to biased outcomes.
The ethical challenge here lies not just in identifying these biases but also in implementing solutions that mitigate them. It requires ongoing monitoring and adaptation of AI systems to ensure they evolve in a direction that promotes fairness and equality. This ongoing process is critical as AI systems are deployed in more areas of public life, affecting an increasingly diverse population.
Bias and Fairness
Addressing bias in AI requires a multifaceted approach. Developers need to be vigilant in selecting diverse and representative training datasets. Additionally, rigorous testing and validation processes must be in place to identify and mitigate biases before AI systems are deployed. This involves not only technical solutions but also ethical considerations, such as ensuring that all groups are fairly represented in the data.
Fair gaming environments, such as those found in a well-regulated casino, serve as a prime example of how AI can be implemented ethically. In these settings, AI algorithms ensure that games are not only engaging but also fair. By utilizing AI to monitor and adjust game mechanics, casinos can maintain a level playing field, preventing any form of manipulation or unfair advantage. This approach ensures that all players have an equal opportunity to succeed, regardless of their background or experience.
The principles of fairness applied in such environments can be translated into other domains where AI is used. For instance, in finance, AI can be employed to ensure that credit scoring systems are free from bias, providing fair access to financial services. Similarly, in education, AI-driven assessment tools can be designed to evaluate students’ performance without discrimination.
Privacy Concerns
AI systems often require vast amounts of data to function effectively. This raises significant privacy concerns, particularly when dealing with sensitive information. Companies must ensure that data collection and processing are transparent and that users have control over their personal information. The challenge lies in balancing the need for data to improve AI systems with the fundamental right to privacy.
To address these concerns, many organizations are adopting privacy-preserving techniques such as differential privacy and federated learning. These methods allow AI systems to learn from data without compromising individual privacy. For instance, differential privacy adds noise to data, making it difficult to identify specific individuals, while federated learning enables AI models to be trained across multiple decentralized devices without sharing raw data. These techniques are becoming increasingly important as AI systems become more pervasive in sensitive areas such as healthcare and finance.
Furthermore, companies must be transparent about their data practices, providing clear information about what data is collected, how it is used, and who has access to it. This transparency is crucial for building and maintaining trust with users, particularly as AI systems become more integrated into daily life.
Accountability and Transparency
A key aspect of ethical AI is accountability. Developers and organizations must be held accountable for the actions and decisions made by their AI systems. This includes implementing robust audit trails and ensuring that AI decisions can be explained and justified. Without accountability, there is a risk that AI systems could be used irresponsibly, leading to harmful outcomes.
Transparency in AI is equally important. Users need to understand how AI systems work and how decisions are made. This transparency builds trust and allows for informed consent. Explainable AI (XAI) is a growing field dedicated to making AI algorithms more interpretable and understandable to humans. By providing explanations for AI-driven decisions, XAI helps users feel more comfortable with the technology, knowing that they can understand and challenge the outcomes if necessary.
Moreover, transparency is essential for regulatory compliance. As governments introduce more regulations governing AI, companies will need to demonstrate that their systems are operating ethically and in accordance with legal requirements. This may involve providing regulators with access to AI systems’ inner workings, ensuring that they are not acting as “black boxes” but rather as transparent, accountable tools.
The Role of Regulation
Governments and regulatory bodies play a crucial role in shaping the ethical landscape of AI. Regulations must balance innovation with public safety and ethical considerations. Policies such as the General Data Protection Regulation (GDPR) in Europe set important precedents for data privacy and AI accountability. These regulations require companies to be transparent about their data practices and to take steps to protect users’ privacy.
International collaboration is also essential. As AI technology transcends borders, harmonized regulations can help ensure that ethical standards are upheld globally. This collaboration can prevent a “race to the bottom,” where countries with lax regulations become havens for unethical AI practices. Global standards can also promote best practices, encouraging countries to adopt ethical frameworks that protect their citizens while fostering innovation.
In addition, governments must invest in education and training programs to ensure that the workforce is prepared for the ethical challenges posed by AI. This includes training developers in ethical AI practices and educating the public about their rights and responsibilities when interacting with AI systems.
Conclusion
The ethical implications of AI are vast and complex, touching every aspect of society. By prioritizing fairness, privacy, accountability, and transparency, we can harness the power of AI responsibly. As we continue to innovate, it is imperative to keep these ethical considerations at the forefront, ensuring that AI benefits all of humanity.
AI’s potential to transform industries and improve lives is immense, but this potential must be realized within an ethical framework that prioritizes the well-being of individuals and society. By working together—across industries, governments, and communities—we can ensure that AI develops in a way that is fair, transparent, and accountable, creating a future where technology serves the greater good.