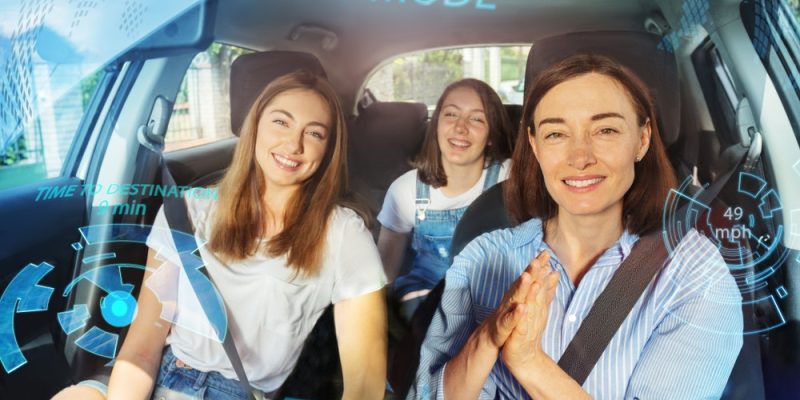
The Role of AI in Autonomous Driving
Autonomous driving is a rapidly evolving technology that has the potential to revolutionize the way we move around in the future. Autonomous cars, also known as self-driving cars, are vehicles that are capable of navigating themselves without human input. These cars rely heavily on artificial intelligence (AI) and machine learning algorithms to make decisions and react to their environment. In this article, we will explore the role of AI in autonomous driving and how it is transforming the automotive industry.
What are Self-Driving Cars?
Autonomous cars are vehicles that use a combination of sensors, cameras, and AI algorithms to navigate themselves without human input. They are designed to sense their environment and make decisions based on that information. These cars can detect traffic signals, pedestrians, other vehicles, and road conditions to safely navigate from point A to point B. There are different levels of autonomy, ranging from Level 0 (no automation) to Level 5 (full automation). Level 5 autonomous cars are completely self-driving and do not require any human intervention.
AI and Autonomous Driving
AI is the driving force behind autonomous driving. Autonomous cars use a combination of machine learning algorithms, computer vision, and deep learning to make decisions and navigate their environment. These algorithms enable the car to analyze vast amounts of data in real-time, allowing it to react quickly and safely to any changes in its surroundings.
What is the Role of AI in Building Infrastructure for Autonomous Vehicles
The success of autonomous driving depends on the infrastructure that supports it. AI plays a crucial role in building this infrastructure. For example, Artificial intelligence can be used to analyze traffic patterns and optimize traffic flow. It can also be used to create detailed maps of roads and highways that are essential for self-driving cars to navigate safely.
How Autonomous Cars Make Life-or-Death Decisions?
One of the biggest challenges facing the development of autonomous cars is how they should make life-or-death decisions. For example, if a self-driving car is in a situation where it must choose between hitting a pedestrian or swerving and potentially harming its passengers, what decision should it make? This is a complex ethical issue that requires careful consideration. AI and machine learning algorithms can help to address this issue by analyzing vast amounts of data and developing decision-making models that prioritize safety.
Is GDDR6 Important for the Future of Autonomous Driving
Graphics Double Data Rate (GDDR) is a type of memory that is essential for autonomous driving. GDDR6, in particular, is becoming increasingly important for self-driving cars. This memory is used to store and process large amounts of data that are generated by the car’s sensors and cameras. GDDR6 is faster and more efficient than previous generations, which means that self-driving cars can process more data in real-time, improving their accuracy and safety.
Autonomous Cars: How Do They Generate their Data
Autonomous cars generate vast amounts of data that are used to make decisions and navigate their environment. This data is generated through a combination of sensors and cameras.
- Sensors: Autonomous cars use a variety of sensors, including lidar, radar, and ultrasonic sensors, to detect their surroundings. Lidar uses laser beams to create a 3D map of the car’s environment, while radar uses radio waves to detect the position and movement of other vehicles. Ultrasonic sensors are used to detect nearby objects, such as parked cars or pedestrians.
- Cameras: Cameras are also essential for autonomous driving. They are used to detect traffic signals, read road signs, and identify objects and pedestrians. Cameras can also be used to create detailed maps of roads and highways, which are essential for self-driving cars to navigate safely.
How Automotive AI Algorithms are Used for Self-Driving Cars
AI algorithms are essential for autonomous driving. They enable the car to make decisions and react to its environment in real-time. There are different types of machine learning algorithms used by self-driving cars, including supervised and unsupervised learning.
Supervised vs Unsupervised Learning
Supervised learning is a type of machine learning algorithm that uses labeled data to train the AI model. In autonomous driving, supervised learning can be used for tasks such as object detection, lane detection, and pedestrian detection. Unsupervised learning, on the other hand, is used for tasks where the data is not labeled. This type of learning is useful for tasks such as anomaly detection and clustering.
What Machine Learning Algorithms are used in Self-Driving Cars
There are several machine learning algorithms used by self-driving cars. These algorithms are designed to perform specific tasks and enable the car to react to its environment. Some of the most popular machine learning algorithms used in autonomous driving include:
AdaBoost for Data Classification
AdaBoost is a machine learning algorithm used for data classification. It works by combining several weak classifiers into a strong classifier. In autonomous driving, AdaBoost can be used for tasks such as object detection and lane detection.
TextonBoost for Object Recognition
TextonBoost is a machine learning algorithm used for object recognition. It works by analyzing the texture of an object and using that information to classify it. TextonBoost is particularly useful for tasks such as pedestrian detection and traffic sign recognition.
Histogram of Oriented Gradients (HOG)
Histogram of Oriented Gradients (HOG) is a machine learning algorithm used for object detection. It works by analyzing the orientation of edges in an image and using that information to detect objects. HOG is particularly useful for tasks such as pedestrian detection and vehicle detection.
YOLO (You Only Look Once)
YOLO is a machine learning algorithm used for real-time object detection. It works by dividing an image into grids and analyzing each grid for objects. YOLO is particularly useful for tasks such as pedestrian detection and vehicle detection.
What Other Learning Algorithms Can Be Used?
There are several other machine learning algorithms that can be used in autonomous driving, depending on the task at hand. These include deep learning algorithms such as Convolutional Neural Networks (CNNs) and Recurrent Neural Networks (RNNs), as well as reinforcement learning algorithms.
Convolutional Neural Networks
Convolutional Neural Networks (CNNs) are a type of deep learning algorithm that are commonly used in autonomous driving. These networks are designed to analyze visual data and are particularly useful for tasks such as object detection and classification. In autonomous driving, CNNs are used to analyze data from cameras and lidar sensors to detect objects such as pedestrians, vehicles, and traffic signs. The network can learn to recognize these objects by analyzing thousands of images, allowing the car to make accurate and informed decisions in real-time. CNNs have also been used to create detailed maps of roads and highways, which are essential for self-driving cars to navigate safely.
Recurrent Neural Networks
Recurrent Neural Networks (RNNs) are another type of deep learning algorithm that are used in autonomous driving. Unlike CNNs, RNNs are designed to analyze sequential data, making them particularly useful for tasks such as predicting future events and trajectories. In autonomous driving, RNNs can be used to predict the behavior of other vehicles and pedestrians based on their previous movements. By analyzing past data, RNNs can predict the future position of these objects and allow the car to make decisions accordingly. This is particularly useful in situations where there is uncertainty, such as at a busy intersection. RNNs can also be used for tasks such as natural language processing, which allows the car to understand and respond to voice commands from passengers.
Final Thoughts
AI and machine learning are driving the development of autonomous driving. These technologies enable self-driving cars to navigate their environment safely and efficiently. As the technology continues to evolve, we can expect to see more advanced AI algorithms being used in autonomous driving.